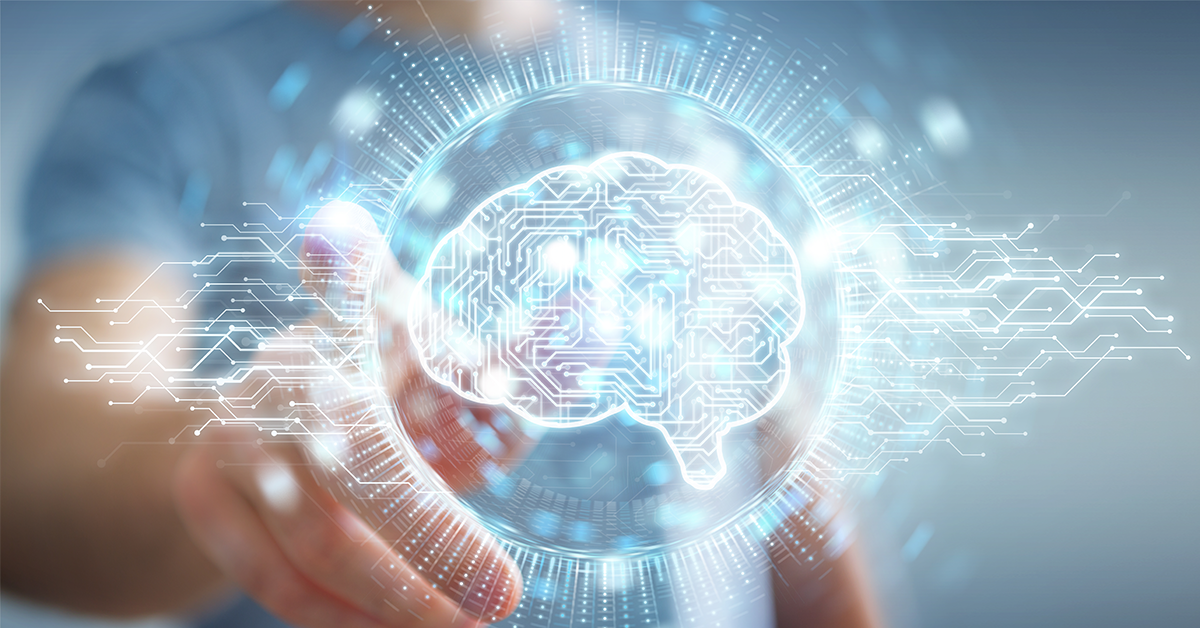
26 Oct Can Computers Predict Suicides Before they Happen?
Suicide is the tenth leading cause of death in the United States, and the numbers have been increasing over the past few decades. Historically, attempts to predict and prevent suicide were based on self-reporting, which can be problematic because people may hide their suicidal thoughts. Relying on single risk factor such as firearms, depression, or substance abuse is only slightly better than chance at making predictions. To achieve noticeable progress in suicide prevention, clinicians need a more accurate way to identify high-risk individuals.
Computers can analyze client data and search for patterns that contribute to individual risk. With the help of machine learning, researchers have studied Electronic Health Records (EHRs), clinical notes, and social media accounts to identify individuals at elevated risk for suicidal behavior.
What is Machine Learning?
Machine learning is a type of artificial intelligence that acquires skills based on self-training and experience rather than explicit programming. Computing systems autonomously discover how to do specific tasks based on training provided by the programmers. In some cases, they can develop better algorithms than a human could.
Supervised machine learning is ideal for risk assessments and scored predictions. With supervised learning, the computer takes labeled data, such as codes in an EHR, then uses the data to create an algorithm that predicts the classification of unlabeled data. For example, programmers provide a large data set of electronic records, some with confirmed suicidal behavior and some without. Then the computer creates an algorithm to predict who is more likely to exhibit suicidal behavior in the future.
Predicting Suicides with EHRs
Multiple factors are at play when it comes to suicide risk, including age, gender, race, and diagnosis. Mental health professionals need a way to aggregate the information and make predictions. Using historical data, researchers from Harvard Medical School showed that computers could predict suicidal behavior 3 – 4 years in advance. Their model assigned scores to EHR codes and then combined them to estimate the cumulative risk for each individual. Not surprisingly, the most highly weighted factors were substance abuse and psychological disorders. However, the model showed that certain injuries, like fractures, and chronic illnesses, such as hepatitis, were also associated with increased suicide rates.
A more recent study from March 2020 looked at EHR data from 3.7 million patients across the U.S. Researchers used supervised machine learning to identify risk factors for suicidal behavior. These results show that developing large-scale risk detection algorithms is possible using existing data. The growing use of EHRs is a powerful tool for mental healthcare providers. With more research, risk scores could be integrated into behavioral healthcare dashboards. However, more research and development is still needed before such tools become a reality.
Natural Language Processing
It’s not just coded data that can raise a red flag on suicidal behavior. Automated tools can analyze narrative notes using a machine learning method called Natural Language Processing (NLP). For example, suicide is more likely among recently hospitalized clients, unfortunately, it’s not feasible to provide interventions for every patient released from the hospital. A team of researchers used machine learning to compare narrative summaries and cause of death in recently discharged patients. The computer searched for 3,000 terms that have subjectively positive or negative meanings (such as lovely or gloomy). The researchers found that NLP modestly improved suicide predictions.
Natural language processing casts a wide net, and it’s less precise than analyzing hard data. A team of researchers in Boston compared diagnostic codes to NLP for suicidal behavior among pregnant women. They found that the use of NLP substantially improves the sensitivity of screening suicidal behavior. However, the process identified many false positives, so the researchers suggest using natural language processing in conjunction with discrete data from EHRs.
Warning Signs on Social Media
Suicide rates among teens and young adults have seen a sharp rise in the past several years. Since this age group frequents social media, it’s an excellent place to reach out. Recent studies show that computers can tell who is at risk and who is not just by analyzing texts and tweets. The technology can search text and flag signs of suicidal thoughts. Social media sites could then generate automated messages with resources such as crisis text lines to those with flagged text.
NLP-based machine learning has the potential to become a powerful screening tool for social media users. Identifying subtle textual cues allows for more responsive suicide prevention. A study published in JMIR Mental Health using NLP compared the use of machine learning on Twitter data to empirically validated measures. The machine learning algorithms identified people at elevated risk with 92% accuracy. The challenges with this type of technology include ethical and privacy concerns. Researchers only screen people who “opt in”, so a fraction of users gets the benefits. The right balance between privacy and prevention is an open question for debate.
Caveats and Resources
Computers are tools to help clinical decision-making, not replace it. Machine learning is still an emerging field, and there will be false positives and negatives as the technology develops. Treatment requires critical thinking to understand the underlying causes. It’s the clinician’s job to decipher computer outputs based on a whole-person perspective.
If you or someone you know is contemplating suicide, call the National Suicide Prevention Lifeline at 1-800-273-8255, or Text 741741 from anywhere in the U.S. to talk with a trained Crisis Counselor. Other resources are also available:
Sorry, the comment form is closed at this time.